Join Date
October 2024
Country
Gender
Not Specified
Age
Not Specified
About Daniel Wigh
Daniel holds a PhD in chemical engineering, and has been working at the intersection of machine learning and chemistry for the past 5 years. In his research, Daniel developed optimization algorithms to aid in designing manufacturing processes for novel pharmaceuticals. Daniel launched ReactWise to democratize access to AI in pharma & biotech with no-code software.
Companies & Work
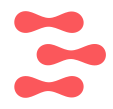
ReactWise
Our Mission:
We aim to accelerate and automate chemical process development by equipping wet-lab chemists with the power of data-driven optimization and robotic execution of experiments.
The Problem:
The discovery of novel pharmaceuticals is one of our most important weapons in fighting disease. However, the drug development pipeline is often held up for many months during the design of chemical processes to manufacture these drugs at scale, delaying FDA trials and lengthening the time until drug launch. Designing chemical processes involves the identification of suitable parameters such as catalyst/temperature/solvent. Currently process development is often done via tedious trial-and-error experimentation (slow) or exhaustive screening (expensive and wasteful).
Our Approach:
In our research, we have developed algorithms for chemical process optimization, which leverage transfer learning and Bayesian optimization. We validated the algorithms in the wet lab, showing an up to 95% reduction in required experiments and cost compared to exhaustive screening. We have made our approaches accessible to chemists through our user-friendly no-code software platform and to automated laboratory equipment with our API.
We aim to accelerate and automate chemical process development by equipping wet-lab chemists with the power of data-driven optimization and robotic execution of experiments.
The Problem:
The discovery of novel pharmaceuticals is one of our most important weapons in fighting disease. However, the drug development pipeline is often held up for many months during the design of chemical processes to manufacture these drugs at scale, delaying FDA trials and lengthening the time until drug launch. Designing chemical processes involves the identification of suitable parameters such as catalyst/temperature/solvent. Currently process development is often done via tedious trial-and-error experimentation (slow) or exhaustive screening (expensive and wasteful).
Our Approach:
In our research, we have developed algorithms for chemical process optimization, which leverage transfer learning and Bayesian optimization. We validated the algorithms in the wet lab, showing an up to 95% reduction in required experiments and cost compared to exhaustive screening. We have made our approaches accessible to chemists through our user-friendly no-code software platform and to automated laboratory equipment with our API.